Artificial Intelligence
Blog
Read the latest from our experts and see what’s new in artificial intelligence, machine learning, and data analytics.
Global Force Management Lessons Learned: Build a Force, Move a Force
Developing an integrated, interoperable, transactional global force management environment that automates workflows, eliminates swivel chair processes and provides risk-informed decision support is critical to the U.S. Army.
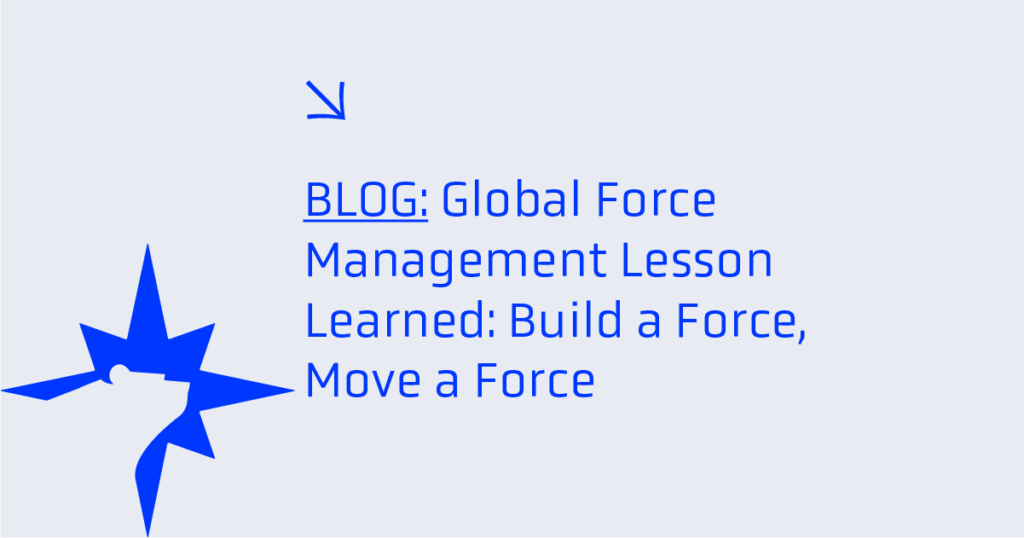
Showing 12 of 124 results
Artificial Intelligence
BigBear.ai's Proactive Stance on AI Safety: Driving a Responsible AI Future in Response to the White House and NTIA
Data Architecture
Data Governance Challenges
Artificial Intelligence
Global Force Management Lessons Learned: Build a Force, Move a Force
Data Architecture
How to Build Fault Tolerant Microservice Using Spring Technology
Data Modeling
The U.S. Navy Wants to Build the World’s First Fully Autonomous Fleet, and BigBear.ai’s Arcas is Helping them Turn a Big Idea into a Reality
ETL
ETL of JSON Data to Neo4j Graph DB
Artificial Intelligence
Is Your Hospital Ready for the “Silver Tsunami”?
Healthcare
How Hospitals Can Prevent Supply Shortages
Healthcare
How the Right Technology Can Help Reduce Average Length of Stay
GFIM
The Force Behind Force Management: Helping the US Army Drive the GFIM Initiative
Healthcare